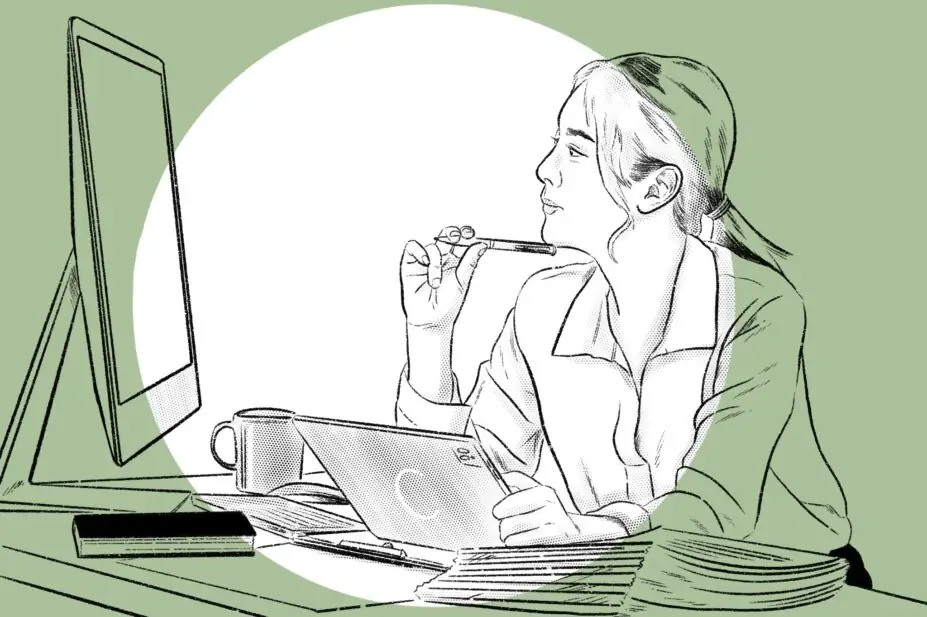
Wes Mountain/The Pharmaceutical Journal
After reading this article, you should be able to:
- Understand where data analytics can be used to support prescribing;
- Have knowledge of common data sources available to both primary and secondary care prescribers;
- Know how to plan and implement a data project.
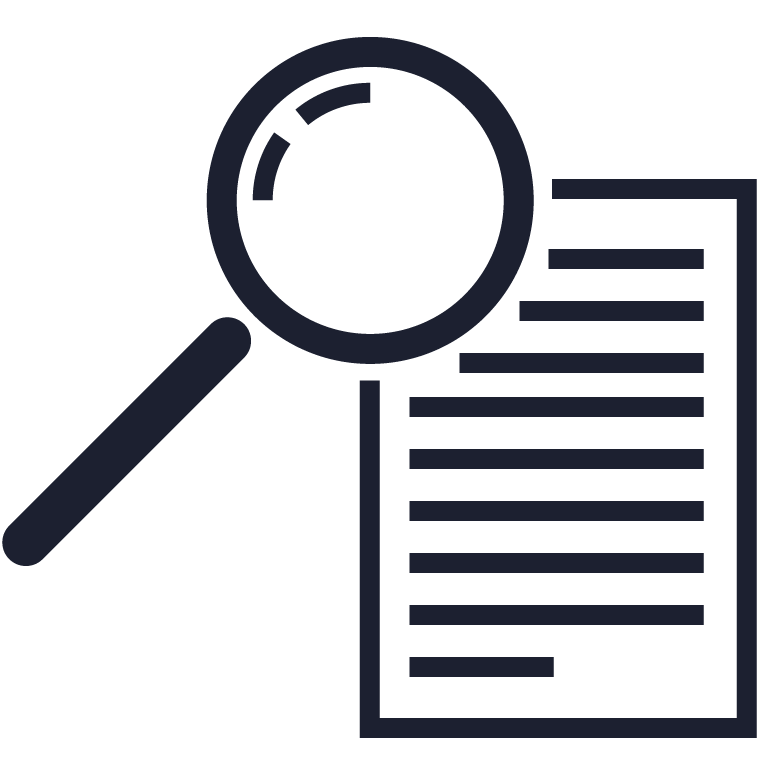
RPS Competency Framework for All Prescribers
This article aims to support the development of knowledge and skills related to the following competencies:
- Domain 2: Identify evidence-based treatment options available for clinical decision making (2.7)
- Accesses, critically evaluates, and uses reliable and validated sources of information.
- Domain 9: Improve prescribing practice (9.3)
- Understands and uses available tools to improve prescribing practice.
Data analytics is the process of analysing data to draw useful conclusions. Insights derived from data have many uses and this is especially true for healthcare.
As healthcare has embraced digital technologies, opportunities for data analysis have increased. Large volumes of data are now generated from systems, such as electronic health records, electronic prescribing systems and pharmacy stock control systems.
Prescribing medications is a complex process with a high degree of risk. To effectively prescribe, a clinician must make decisions on an individual patient level, in addition to having an appreciation of the effects of their prescribing practices on wider population health and economics.
This article will explore some of the ways that data analytics can be used to optimise prescribing practices and demonstrate how to plan a data analytics project. To help further expand your prescribing skills, additional related articles are linked throughout. You will also be able to test your knowledge by completing a short quiz at the end of the article.
Using data to identify variation in prescribing practice
One application of data analytics within prescribing is to identify variation in prescribing practice. If a variation can be first identified and then explored, it can lead to efficiency improvements, cost reductions, improved medicines safety and medicines optimisation.
Despite the availability of extensive national and local guidance, unwarranted variation in prescribing practice continues to exist between UK healthcare settings. The factors that can introduce unwarranted variation to prescribing are complex; they include an individual prescriber’s level of experience, or the amount of education and training they have received, and societal factors, such as pressure from patients to prescribe certain medicines[1]. Variation in prescribing practice can have negative consequences for patients and healthcare systems — for example, variation could indicate that patients are receiving inappropriate supplies or course lengths of antibiotics, are at increased risk of adverse events, or are failing to gain access to improved treatment options[2].
Medicines optimisation focuses on the value that medicines deliver to patients, ensuring that they are both cost and clinically effective. To provide the best outcomes for patients, prescribers should be able to recognise opportunities for medicines optimisation[3]. There are many examples of where data analytics can help to identify these opportunities.
Increasing the appropriateness of prescribing
Ensuring that prescribing is appropriate is one of the main aims of medicines optimisation. There are several resources available to help pharmacists reduce inappropriate prescribing.
In primary care, tools such as OpenPrescribing can identify variations in prescribing practices. For example, it can show the variations in opioid prescribing across different regions in England and link increased use to factors such as social deprivation[4]. Awareness of this data can help prescribers to identify patients at risk and inform their approach to consultations; for more on this topic, see ‘Factors influencing communication when prescribing’. OpenPrescribing collates NHS primary care prescribing data at an individual practice level and makes it accessible to clinicians. The website provides free access to both predefined and custom reports. For instance, users can produce reports on the prescribing of broad-spectrum antibiotics, the supply of anxiolytics per 1,000 patients, or items supplied which should not be routinely prescribed in secondary care[5]. OpenPrescribing has been shown to a have positive impact on prescribing practices, particularly when used to identify cost-saving opportunities[6].
In secondary care, there are several opportunities for identifying areas for prescribing improvements. Many electronic patient record or electronic prescribing systems can provide data that highlight prescribing variations. Data can often be obtained through direct interrogation of hospital systems using business intelligence software. Some trusts will also have access to third-party systems. RX-Info has several programs that provide insights into medication supply and how this differs between specialities within a trust. It is also able to draw comparisons between other trusts of a similar size. Tools such as these can identify areas of prescribing variation that require further investigation, such as the variation in use of broad-spectrum antibiotics on different wards.
Reducing inappropriate polypharmacy
Polypharmacy can become problematic when previously prescribed medications are no longer indicated or pose a risk to the patient through interactions or adverse effects[7]. Tackling problematic polypharmacy forms a large part of medicines optimisation and an effective prescriber should recognise and take opportunities to reduce it.
Data-led opportunities to target polypharmacy can be found in all settings. For primary care, ePACT2 (provided by the NHS Business Services Authority) is a business intelligence tool that allows users to analyse prescribing data and spot trends. Polypharmacy prescribing comparators provided by the tool can help identify patients at risk of problematic polypharmacy, such as those prescribed multiple analgesic medicines at any one time[8].
In secondary care, there are also many opportunities to use analytics to tackle polypharmacy. The ability to retrieve data from electronic prescribing systems means that patients on certain treatments can be quicky and easily identified. For example, it is possible to produce live reports of current inpatients on high-dose proton-pump inhibitors or on multiple laxatives or opioid analgesics. This type of data analysis can provide input for targeted reviews of inpatients who may not necessarily be reviewed otherwise.
Medicines safety improvements
Data analytics can also be used to address wider medicines safety issues and identify prescribing trends that may need to be addressed. The NHS Business Services Authority has a medicines safety dashboard, displaying a set of prescribing indicators linked to admission data, such as patients aged over 65 years prescribed a non-steroidal anti-inflammatory and admitted to hospital with a gastrointestinal bleed. Teams can use the tool to review prescribing at a local level.
Skills required for data analysis
There are a range of skills required to carry out effective data analysis, dependent on the scope of the research question. As a prescriber, it is important that you invest in the development of these skills, which can be acquired from freely available online courses and tutorials, qualifications from formal education settings and learning from peers and colleagues in the workplace.
To illustrate some of the skills and approaches required for effective use of data when prescribing, the following section provides a basic outline of how a typical data analytics project is conducted.
Planning a data analytics project
Step 1: Choosing a model
Applying a structured approach to designing a data analytics project will help to ensure its success. One of the most popular methods is the Cross Industry Process for Data Mining (CRISP-DM)[9]. It describes the typical phases of any data analytics project and provides a useful framework.
The CRISP-DM process is iterative (see Figure 1)[10]. A good level of domain knowledge in the subject area being analysed and a thorough understanding of the problem being addressed will inform the requirements of the data needed. Healthcare data can be challenging to collect and analyse without an understanding of underlying drivers and situational context. For example, a project analysing antibiotic course lengths would require an understanding of the principles of intravenous to oral switches. Data is collected, cleaned and refined, ready for analysis. Analysis of the data may well inform further understanding of the problem and drive the need for further analysis.
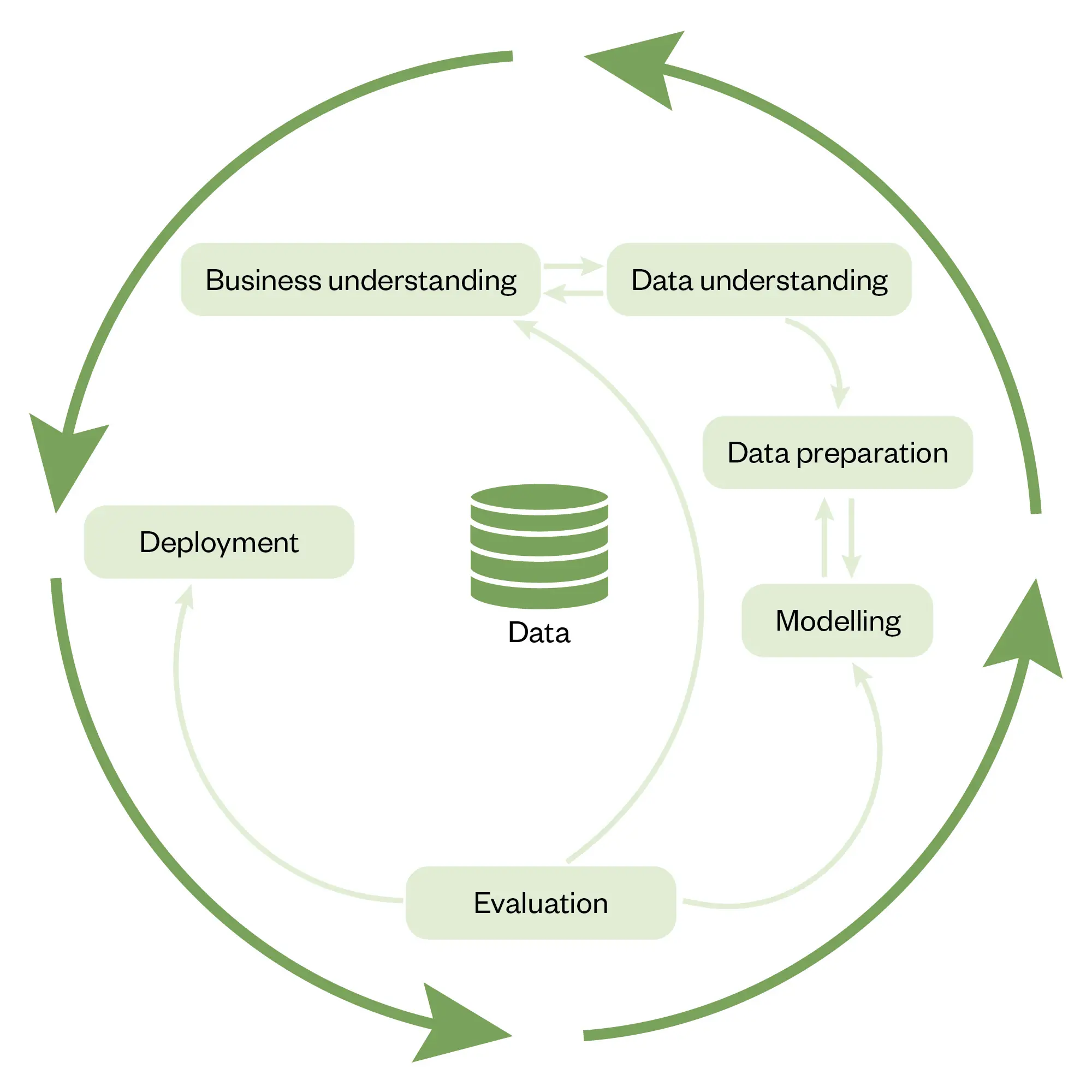
The Pharmaceutical Journal
Step 2: Gathering data
The rise of digital systems in healthcare has led to the creation of large and complex datasets, which provide much opportunity for analysis. Large datasets are often described as ‘big data’. The characteristics of big data can be described using the ‘three Vs’: volume, velocity and variety. ‘Volume’ refers to the amount of data, ‘velocity’ describes the speed at which that data is produced and ‘variety’ describes the different types of data. These three factors can drastically affect the approach needed for a project, depending on their nature; for example, poorly standardised data with lots of variation will require more time to prepare to produce meaningful results[11]. Although prescribing data is often standardised, this is not always the case and data quality can vary greatly.
There are several resources that can be used to gather prescribing data:
- NHS Business Services Authority, which publishes several freely accessible prescribing and dispensing datasets;
- OpenPrescribing, which provides tools to analyse the NHS datasets;
- PRESQiPP, which provides access to prescribing analytics in primary care (however, it requires additional access granted to medicines management teams);
- RX-Info packages are available in most secondary care providers that give the user access to medication supply data that can be compared between wards, directorates and other trusts;
- Other techniques to obtain data, which include taking data directly from the source. Many electronic prescribing systems, for example, will have an associated business intelligence software that allow a user to extract data. This may require specialist knowledge of database structures and structured query language and will have/need appropriate governance rules;
- Audit tools, which can be designed to collect data electronically. Tools such as Microsoft Forms can be used to design input forms, which will allow for standardised data entry, helping to reduce effort required for analysis.
Step 3: Applying analytical skills
A variable is a defined attribute or object of a study and can be either quantitative or categorical (see Figure 2). Choosing which variables to include in a dataset will influence the success of any analysis.
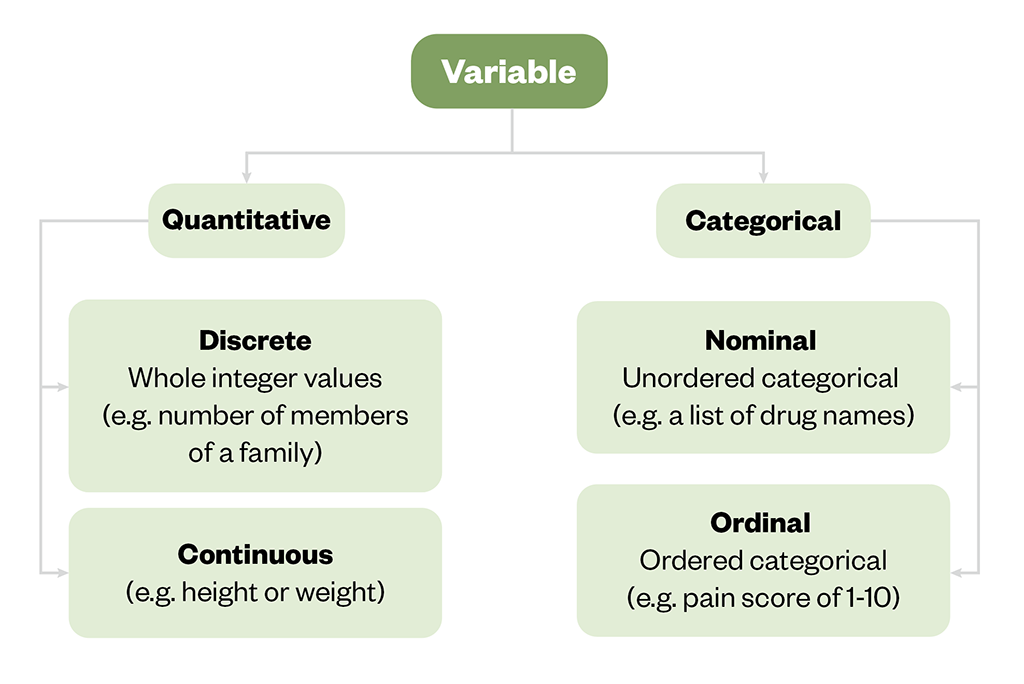
The Pharmaceutical Journal
An understanding of which statistical test to use when drawing conclusions from data will improve the meaningfulness of results.
There are two main areas of statistics: inferential and descriptive. Inferential statistics estimates whether patterns found in data are likely to be present in a wider population, but descriptive statistics (see Box) will be sufficient for many projects.
Box: The three main types of descriptive statistics
- Mean: a simple average of two or more numbers. Easy to calculate by adding all figures in a dataset and dividing by the number of figures present. It is highly susceptible to being affected by outliers in a dataset;
- Median: the middle value in a sorted list. The median is preferred where there are outliers in a dataset;
- Mode: the number which appears most frequently in a dataset.
The case study at the end of the article illustrates how these principles can be used in a practice example. For more on this topic, see ‘A basic introduction to statistics’.
Data analysis tools
A range of data analysis tools are available that can enable you to interrogate data in different ways. The type of tool used will depend on the nature of the data analysis project. It is beyond the scope of this article to explore data analysis tools in detail but some commonly used examples are summarised below.
Microsoft Excel
Excel is a spreadsheet editor and one of the most common tools used for data analysis. It is suitable for most data manipulation tasks.
BI software
There are multiple business intelligence (BI) software platforms available to help with analysing data. Examples include Microsoft Power BI, Tableau or Qlik Sense. These products can be very useful and are often easier to use than computer coding options. However, they are not as accessible as Excel, as they often require a paid subscription license.
Structured query language
Structured query language (SQL) is a query language used to extract information from relational databases, which store information in tables of rows and columns, similar in structure to an Excel worksheet. An understanding of SQL may be needed to extract information from some systems and is common with some electronic patient record or electronic prescribing systems. There are many resources available online that teach the basics of SQL.
Python and R
Python and R are two programming languages that are popular for data analytics. Python is a general purpose language that, in addition to data analytics, can be used to build websites and desktop applications. R is specifically created for statistical computing. Both are widely used in data science and academia owing to their functionality. They also have a wide support community including the NHS-R and NHS Python communities. To learn more about either language, there are many online tutorials available.
Step 4: Presenting data and measuring change
The impact of a data analytics process is unlocked when the results lead to conclusions that prompt change or improvement, or confirm that an intervention is achieving its intended outcomes. This is more easily achieved when data are presented in simple and easily understandable formats. For the purposes of process improvement, stakeholders will want to be able to visualise trends and variation over a prolonged period.
Statistical process control (SPC) charts are one method that can be implemented to visualise variation in data plotted over time and are commonly used throughout the NHS[12]. In Figure 3, the data have been calculated for 12 months and presented in an SPC[13]. The representation shows that course length has decreased towards the target of 5 days during this time. SPC charts plot upper and lower control limits, which make it easy to identify out-of-range values.
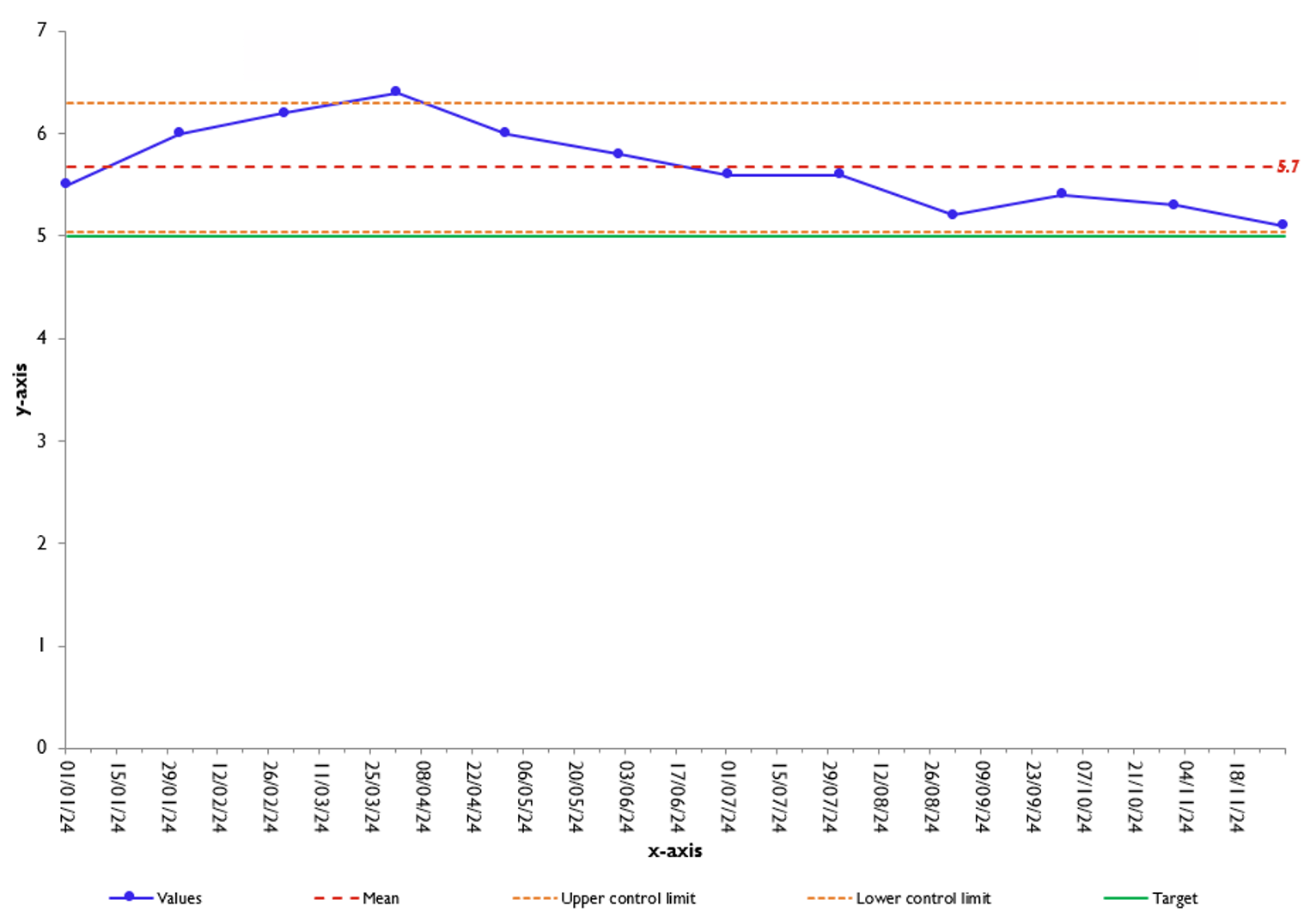
The Pharmaceutical Journal
Any further interventions that are made to improve prescribing processes will also need to be tested, highlighting the need to design projects that are easily repeatable and allow for comparison.
Case in practice: a data project to improve antimicrobial stewardship
While working on a respiratory ward in an acute hospital trust, you have introduced several measures to improve antimicrobial stewardship, including providing feedback to prescribers and making prescribing guidance more accessible. To demonstrate the impact of the improvements, you have decided to audit the quality of prescribing in community-acquired pneumonia.
You have obtained a dataset of antibiotic prescribing and would like to compare the quality of prescribing across two months.
Calculating the mean course length
Month 1: (5 + 5 + 7 + 21) / 4 = 9.5 days
Month 2:(5 + 7 + 7 + 5) / 4 = 6 days
This simple test shows that prescribing has potentially improved between months 1 and 2; however, as described earlier, the mean is susceptible to outliers. The value of 21 days in month 1 has increased the value of the mean, so that it is not representative of typical prescribing practices. This also demonstrates the value of data preparation and cleaning. Domain knowledge and understanding of antimicrobial prescribing would probably have prompted you to question these results prior to data analysis. It is likely that the course length has been incorrectly recorded or the indication has been documented incorrectly.
Calculating the median course length
In a list of even numbers, the median is the mean of the two middle numbers after the number has been assorted in order.
Month 1: [5,5,6,21] = 5.5 days
Month 2: [5,5,7,7] = 6 days
In this example, the result has not been drastically affected by outliers. The median has provided a value that is more representative of the dataset and has not been skewed by the outlier value. This demonstrates the importance of understanding what is the most appropriate statistical test to apply.
Summary
The introduction of digital systems into healthcare has led to the creation of widespread opportunities to obtain and analyse data that provide useful insights into patient care. This article has introduced some of the basic principles of data analysis and provided some indicative examples of how data can be used to improve prescribing practice. Pharmacist prescribers as experts in medications are well placed to be at the forefront of prescribing data analytics. There is no limit to the number of ways prescribers can use data to explore different clinical questions, and developing skills in data analysis should form an important component of ongoing professional development as they look to expand their scope of practice and drive improvements in patient outcomes.

Knowledge check

Expanding your scope of practice
The following resources expand on the information contained in this article:
- FutureNHS Workspace: Analyst X — a collaboration platform providing opportunities to collaborate, share and learn about data science.
- Future Learn: Health Data and Analytics — an online course that explores key concepts in data analytics, systems theory and information governance, and applies them to healthcare decision making.
There are also many free general data analytics courses available online (easily found through search engines).
- 1Carter M, Chapman S, Watson MC. Multiplicity and complexity: a qualitative exploration of influences on prescribing in UK general practice. BMJ Open. 2021;11:e041460.
- 2Devine P, O’Kane M, Bucholc M. Trends, Variation, and Factors Influencing Antibiotic Prescribing: A Longitudinal Study in Primary Care Using a Multilevel Modelling Approach. Antibiotics. 2021;11:17.
- 3Medicines optimisation. NHS England. 2024. https://www.england.nhs.uk/medicines-2/medicines-optimisation/ (accessed June 2024)
- 4Curtis HJ, Croker R, Walker AJ, et al. Opioid prescribing trends and geographical variation in England, 1998–2018: a retrospective database study. The Lancet Psychiatry. 2019;6:140–50.
- 5Explore England’s prescribing data. OpenPrescribing. 2024. https://openprescribing.net/ (accessed June 2024)
- 6Walker AJ, Curtis HJ, Croker R, et al. Measuring the Impact of an Open Web-Based Prescribing Data Analysis Service on Clinical Practice: Cohort Study on NHS England Data. J Med Internet Res. 2019;21:e10929.
- 7Polypharmacy: Getting our medicines right. Royal Pharmaceutical Society. 2024. https://www.rpharms.com/recognition/setting-professional-standards/polypharmacy-getting-our-medicines-right (accessed June 2024)
- 8Medicines optimisation – polypharmacy. NHS Business Services Authority. 2024. https://www.nhsbsa.nhs.uk/access-our-data-products/epact2/dashboards-and-specifications/medicines-optimisation-polypharmacy (accessed June 2024)
- 9Schröer C, Kruse F, Gómez JM. A Systematic Literature Review on Applying CRISP-DM Process Model. Procedia Computer Science. 2021;181:526–34.
- 10Kelleher J, Tierney B. Data Science. MIT Press 2018.
- 11Diez D, Cetinkaya- Rundel M, Barr C. OpenIntro Statistics Fourth Edition. OpenIntro. 2019. https://www.biostat.jhsph.edu/~iruczins/teaching/books/2019.openintro.statistics.pdf (accessed June 2024)
- 12Statistical process control tool. NHS England. 2024. https://www.england.nhs.uk/statistical-process-control-tool/ (accessed June 2024)
- 13SPC chart template. AQUA. 2024. https://aqua.nhs.uk/resources/spc-chart-template/ (accessed June 2024)