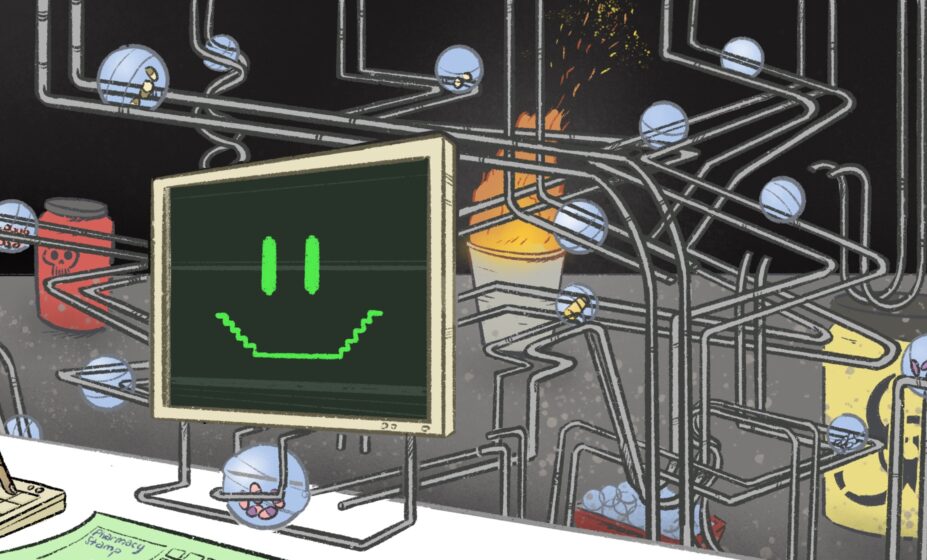
Wes Mountain/The Pharmaceutical Journal
The rash of artificial intelligence (AI) chatbots breaking out across our screens has got everyone poking and prodding at them and wondering — is this something I need to see a doctor about?
The unrelenting pace of AI advancement has rapidly shifted to place healthcare at the centre of the developers’ crosshairs. AI chatbots are computer programs that use a branch of AI called natural language processing (NLP) to understand human language, allowing them to interpret questions, automate responses and simulate human conversations. The hope is that, in healthcare, these cutting-edge technologies will revolutionise patient care, boosting productivity and streamlining day-to-day operations.
Medical chatbot buzz started in February 2023 when Open AI’s ChatGPT 3.5 was found to pass the US Medical Licensing Exam (USMLE) with similar scores to the average human, despite no field-specific training[1]. By June 2023, Google’s medically tailored model — Med-PaLM 2 — outdid ChatGPT’s score by more than 25 percentage points and outperformed doctors at answering patient questions[2,3].
Everyone was completely astonished
Benedict Morath, a pharmacist at Heidelberg University Hospital
Headlines like these led hospital pharmacist Benedict Morath and colleagues at the Heidelberg University Hospital in Germany to put the performance of ChatGPT 3 to their test — on drug information[4].
“As pharmacists, we need to think about how this will affect our practice,” says Morath.
“We said, let’s make this a realistic scenario, let’s take the questions we hear every day. Let’s take 50 [questions] because this would reflect a normal workload of two working days,” he outlines. “Simple ones by physicians and nurses, but also more complex ones because we have an affiliated drug information centre in our hospital pharmacy, and we are doing ward rounds and [patient] counselling.”
Going in with low expectations, “everyone was completely astonished”, Morath says.
“Thirteen questions were correct, you could have used them in practice, and they were sometimes quite complex.”
However, the team noted that correct answers tended to be more readily available in drug labels or in summaries of product characteristics, while poor answers were to questions that often required individualised literature searching, such as dosing for patients on dialysis. Assessing the answers by content accuracy, risk of patient harm and ability to generate a patient management action, answers to a total of 37 out of 50 questions were deemed unfit for use, with 13 of these deemed high-risk for patient harm; for example, giving incorrect dose equivalency information for diabetes drugs glibenclamide and glimepiride, which could lead to dosing errors[4].
Hallucination trap
The danger of the AI chatbot ‘hallucination’ phenomenon — which is where the chatbot produces answers that are factually incorrect but feel convincing owing to the style and tone they are presented in — was also concerning.
“When we asked questions about aminoglycosides dosing in obesity, it provides formulas, it looks like it calculates it, it really looks like an expert, but it’s complete nonsense. This was really frightening,” Morath explains.
The hallucination trap comes from the underlying AI system, known as a ‘large language model’ (LLM). Purposefully designed to analyse language syntax and produce an output that mimics the dataset it was trained on, LLM-generated content might look right — but it has a critical flaw.
It’s a generative model — we must not confuse this with knowledge
Rebecca Pope, digital and data science innovation lead at Roche UK
“It has no idea if it’s correct,” explains Rebecca Pope, digital and data science innovation lead at Roche UK, whose role involves working with the NHS, governments, regulators, clinicians and patients on how advanced technologies, such as AI, can be brought into healthcare. “It’s a generative model — we must not confuse this with knowledge.”
Traditional AI is predictive, which means it is designed to make accurate deductions from data, whereas generative AI is probabilistic, meaning the LLM will instead look at the probabilities of words being used together to form its response.
“You’ll give it a prompt, so: ‘Given what you’ve learnt, for example, trawling through data on the internet, tell me what I should prescribe next for this kind of patient?’ And actually, what it will do, is it will go: ‘Probabilistically, in the data, I’ve seen this patient has this kind of diagnosis and very often drug ‘X’ is prescribed’ — so it will suggest ‘X’ as the prescribed medication. That’s what it is actually doing,” explains Pope.
Combined with the sheer size of these models — GPT 3 has 170 billion parameters or ‘connections’ — the leap from source to answer is nearly impossible to trace or validate[5].
“We think that models like ChatGPT 4, PaLM, PaLM 2 are very strong intelligence substrates, but using them in a safety critical domain like medicine requires specialised fine-tuning,” says Vivek Natarajan, research lead for LLMs in medicine at Google Research.
“And that is why we built out Med-PaLM, where we put in some more medical domain data for fine-tuning and gave them a lot of expert demonstrations around how to answer medical domain questions properly.”
Med-PaLM and Med-PaLM 2 subject an LLM to further training using smaller, curated sets of medical information and expert demonstrations. The expert demonstrations include example questions and answers with step-by-step details of the underlying medical reasoning process from expert clinicians. The team also used a technique called ‘ensemble refinement’, where the LLM generates multiple answers and learns from self-evaluation which answer was the ‘best’ one[2,6,7].
Setting ‘guardrails’
Innovation in these types of fine-tuning techniques is aimed at building increasingly accurate models, but this approach alone cannot fully bridge that leap from source to answer — or the chasm in clinician trust it leaves.
Lacking a guarantee of accuracy, LLM chatbots require extensive human-led quality assurance on their output.
“You need to do prompt engineering techniques with humans in the loop to identify the rabbit holes you want to close off and not allow the models to go down,” explains Brian Anderson, chief digital health physician at US-based non-profit Mitre, where he leads digital health research initiatives across industry and government.
Prompt engineering is a technique that stress-tests the model by asking it questions in as many variations as possible to try to catch ‘wrong’ responses — anything from incorrect ‘hallucinations’ to racist or misogynistic language[8,9]. Instructions are then coded into the model as ‘guardrails’ to prevent the chatbot from producing these wrong answers again.
“It’s a long process of assuring the guardrails are addressing any kind of edge case [or ‘unusual question’]. And that’s the risk too — it’s really hard to identify all potential edge cases.”
But are guardrails appropriate for clinical uses?
With these AI patients, I can make sure that all the common scenarios that you will see in a community-based clinic are programmed in
Jeff Nagge, clinical associate professor, School of Pharmacy, University of Waterloo
One person making it work is clinical associate professor Jeff Nagge at the University of Waterloo’s School of Pharmacy in Ontario, Canada, who masterminds a training course for community pharmacists. The course includes a virtual anticoagulation clinic that incorporates ChatGPT 4 to power patient ‘personas’ for realistic patient-pharmacist interaction[10].
“With these AI patients, I can make sure that all the common scenarios that you will see in a community-based clinic are programmed in. So, you’ll see someone with an extremely elevated INR [international normalised ratio], you’ll see someone just starting warfarin, you’ll see some drug-drug interactions,” Nagge describes.
Opening geographical barriers, standardising trainee experiences, and bridging the gap between book learning and real-life experience, the virtual clinic has landed well with “wonderful feedback” — so much so that Nagge is working on an antihypertensives course next.
But its success is heavily down to Nagge’s personal expertise and commitment.
“I spent probably at least three weeks, more days than not, at least five hours a day, going through and trying to break my characters. What if I say this, what if I say that?” Without Nagge’s custom-made guardrails, the model might generate patient-scenarios that are too complex for the automated grading to properly assess — giving false security to a trainee or imparting flawed clinical judgement.
While it might work for such tightly scoped training use-cases, broader or complex clinical uses are a different matter. Would you be happy that your insulin was produced under a guardrail?
“I’m not a fan of ‘guardrails’,” Pope puts it bluntly. “For me, it’s a really bad word. Because, for me, it enables flexibility that doesn’t protect people in my view. I think it should be highly regulated.”
Relying on retrospective identification of wrong answers could be too much like playing ‘whack-a-mole’ with risk for comfort. But regulation is struggling to keep up.
Use-case-centric regulation
“Generative AI blew up the ‘traditional’ AI technology-focused approach to regulation of AI,” says Stefan Harrer, AI ethicist and chief innovation officer at the Australia-based Digital Health Cooperative Research Centre.
Previously, regulation centred on certain ‘principles’ — such as the model’s explainability, transparency, testability and fairness. But, because generative AI has billions of parameters and is difficult to validate, it starts on the back foot for much of these criteria.
The UK’s AI approach is outlined in ‘A pro-innovation approach to AI regulation’ white paper, most recently updated in June 2023, which sticks to traditional principles-based assessment. In the paper’s ministerial foreword by Michelle Donelan, secretary of state for science, innovation and technology, she says the policy is “deliberately designed to be flexible” and adjust “as the technology evolves”, acknowledging the different flavours of AI and the varying risks associated with their use[11].
The trail blazer [for AI regulation] is the EU with its AI Act
Stefan Harrer, chief innovation officer, Digital Health Cooperative Research Centre
However, Harrer points out that assigning a level of risk to a generative AI model is particularly difficult, “because the use cases are so diverse”. For example, the same LLM could be used to generate patient discharge summaries, or recommend a treatment plan, which have different risk levels.
“The trail blazer is the EU with its AI Act,” Harrer continues, noting that it takes a use-case-centric approach, regulating models based on “the specific application and the outcomes of using AI, and not what type of AI is actually being used”[12].
With the repositioning of the original big-brand LLMs as customisable platforms for hire — such as ChatGPT underpinning Nagge’s training programme, or how Med-PaLM 2 is selectively available on Google Cloud to “some of the biggest companies in the world”, says Natarajan — use-case-centric regulation seems like a neat option to let LLM innovation continue apace while preventing unregulated real-world application[13].
The Coalition for Health AI (CHAI) — a US-based community of academic health systems, organisations and expert practitioners of AI and data science co-founded by Anderson — is already exploring the next steps and what this might look like in practice.
“What we’re trying to do at CHAI is have a consensus-driven agreement on what assurable AI, and specifically in this space, in LLMs means,” Anderson explains. CHAI’s blueprint for trustworthy AI recommends the creation of ‘AI assurance labs’ to help deliver the independent verification of AI tools (see Box)[14].
“We envision supporting this ecosystem of assurance labs with a technical practise manual that would enable the assurance labs to have a framework to independently, transparently, evaluate models for adherence to those standards.”
Box: The Coalition for Health AI’s blueprint for AI in healthcare
Useful: The concept of usefulness covers a range of criteria — AI tools have to first be usable (e.g. easy to use and integrated into clinical workflow). They must be testable to determine that they deliver a meaningful benefit; and their output should be validated and proven to be reliable and reproducible. This must all be monitored throughout use, with an appropriate mechanism for reporting errors.
Safe: AI tools must show ‘non-inferiority’ — at the very least, be no worse for patient outcomes than the status quo. This covers output accuracy, the user interface, such as with ‘automation bias’ (uncritical acceptance of automated recommendations), but also principles of fairness and inequity (differential outcomes within patient groups, e.g. age, ethnicity, sex).
Secure: To be fit for real-life use, AI tools must be resilient against change in use, and privacy-enhanced to protect patient data and clinical confidentiality.
Accountable, transparent, explainable and interpretable: An early emphasis was placed on the need for traceability from source data to output, so it could be explained and more readily interpreted for clinical use. However, with LLMs essentially a ‘black box’ lacking these features, CHAI is rethinking what these principles mean for generative AI.
Bias and fairness
It remains to be seen if these measures will bring sufficient scrutiny to generative AI in healthcare to satisfy clinicians, but not everyone is convinced.
Reporting requirements and regulatory guidance do not go far enough in requiring evaluation of equity or bias
Matthew DeCamp, associate professor of medicine, University of Colorado
“It’s still early stage, but my view is that reporting requirements and regulatory guidance do not go far enough in requiring evaluation of equity or bias,” says Matthew DeCamp, associate professor of medicine at the University of Colorado.
“Requirements tend to look at population level outcomes — does it work, is it safe? And what we’re calling attention to its differential impact, requiring evaluation of performance across different sub-groups.”
Bias and fairness in AI are normally considered in terms of the underlying data but chatbots bring another element into the mix.
Seemingly inconsequential aspects of a chatbot, such as the choice of avatar looks, gender, race, age, style and speech, could help patient engagement by improving medicines adherence or encourage sharing in socially ‘taboo’ areas such as sexual health. But it is equally possible a patient may not connect with an avatar’s features, undermining engagement. There is simply not enough research from which to draw any conclusions — another point DeCamp’s team call attention to, and an aspect to be taken seriously within AI product design regardless.
There’s also an argument that LLMs and medical chatbots put the cart before the horse. Natural language processing (NLP) —the technology family to which LLMs belong‚ could be targeted at more mundane problems, such as translating clinical notes into ICD-10 codes to make NHS reimbursement claims easier or writing patient discharge summaries.
“There are things we can be doing in the NLP space that are decoupled from LLMs but hugely impactful for productivity, patient care and outcomes, but we’re not doing [them], because like magpies, we’re getting all excited about the glittery thing,” Pope states.
“To truly develop and understand if this can be brought into healthcare at scale, is why Roche has partnered with Great Ormond Street Hospital (GOSH),” she says. In this first-of-its-kind collaboration, Roche UK is providing funding and staff to work closely with GOSH’s data research, innovation and virtual environments (DRIVE) unit, looking to co-develop digital solutions for the hospital, including NLP tools, that have genuine impact for clinicians[15].
“How hard is it to bring these technologies into hospital? What are the limitations, whether that be infrastructure, computer power, workforce, or clinical buy-in?” Pope asks.
Roche is not the only one keen to enter this space; Microsoft has partnered with electronic health record provider Epic to leverage OpenAI’s technology on these data, searching for efficiency and productivity gains.
But another big name is suspiciously absent.
“I’m still waiting for it,” says Harrer. “Amazon has been interestingly quiet in all of this, but what I see is a digital pharmacy that will tap, probably quite substantially, into the efficiency-boosting powers of generative AI by integrating tools in the workflow there.”
Speculation — and possibility — is running riot. But clearly it is no longer a case of ‘if’ for AI in healthcare, but ‘when’, and how best pharmacy can harness it.
- 1Kung TH, Cheatham M, Medenilla A, et al. Performance of ChatGPT on USMLE: Potential for AI-assisted medical education using large language models. PLOS Digit Health. 2023;2:e0000198. doi:10.1371/journal.pdig.0000198
- 2Singhal K, Tu T, Gottweis J, et al. Towards Expert-Level Medical Question Answering with Large Language Models. 2023. doi:10.48550/ARXIV.2305.09617
- 3Ayers JW, Poliak A, Dredze M, et al. Comparing Physician and Artificial Intelligence Chatbot Responses to Patient Questions Posted to a Public Social Media Forum. JAMA Intern Med. 2023;183:589. doi:10.1001/jamainternmed.2023.1838
- 4Morath B, Chiriac U, Jaszkowski E, et al. Performance and risks of ChatGPT used in drug information: an exploratory real-world analysis. Eur J Hosp Pharm. 2023;:ejhpharm-2023-003750. doi:10.1136/ejhpharm-2023-003750
- 5Brown TB, Mann B, Ryder N, et al. Language Models are Few-Shot Learners. 2020. doi:10.48550/ARXIV.2005.14165
- 6Singhal K, Azizi S, Tu T, et al. Large Language Models Encode Clinical Knowledge. 2022. doi:10.48550/ARXIV.2212.13138
- 7Raieli S. Google Med-PaLM: The AI Clinician. Medium. 2023.https://towardsdatascience.com/google-med-palm-the-ai-clinician-a4482143d60e (accessed 8 Aug 2023).
- 8Cohen J. Right on Track: NVIDIA Open-Source Software Helps Developers Add Guardrails to AI Chatbots. NVIDIA . 2023.https://blogs.nvidia.com/blog/2023/04/25/ai-chatbot-guardrails-nemo/ (accessed 8 Aug 2023).
- 9Wang Y, Singh L. Adding guardrails to advanced chatbots. 2023. doi:10.48550/ARXIV.2306.07500
- 10Madzarac M. Generative AI: ChatGPT enhances experiential learning in pharmacy. University of Waterloo. 2023.https://uwaterloo.ca/artificial-intelligence-institute/news/generative-ai-chatgpt-enhances-experiential-learning (accessed 8 Aug 2023).
- 11Department for Science, Innovation and Technology, Office for Artificial Intelligence. A pro-innovation approach to AI regulation. Gov.uk. 2023.https://www.gov.uk/government/publications/ai-regulation-a-pro-innovation-approach/white-paper (accessed 8 Aug 2023).
- 12EU AI Act: first regulation on artificial intelligence. European Parliament. 2023.https://www.europarl.europa.eu/news/en/headlines/society/20230601STO93804/eu-ai-act-first-regulation-on-artificial-intelligence (accessed 8 Aug 2023).
- 13Gupta A, Waldron A. A responsible path to generative AI in healthcare. Google Cloud. 2023.https://cloud.google.com/blog/topics/healthcare-life-sciences/sharing-google-med-palm-2-medical-large-language-model (accessed 8 Aug 2023).
- 14CHAI Unveils Blueprint for Trustworthy AI in Healthcare. CHAI. 2023.https://coalitionforhealthai.org/updates/april-4th-2023 (accessed 8 Aug 2023).
- 15GOSH and Roche UK partnership using AI to bring personalised healthcare to children. Great Ormond Street Hospital for Children NHS Foundation Trust. 2023.https://www.gosh.nhs.uk/news/gosh-and-roche-uk-partnership-using-ai-to-bring-personalised-healthcare-to-children/ (accessed 8 Aug 2023).
2 comments
You must be logged in to post a comment.
Worrying. So many things could and will go wrong. Throw in bad actors and the dangers could be immense. International regulation and expert overseeing is needed early days or sleepwalking into a real mess could ensue.
The article mentions the risk of AI chatbots providing incorrect information, but it could be expanded to include a more in-depth discussion of the ethical considerations involved in using AI in healthcare.
https://www.technobridge.in/clinical-research-course