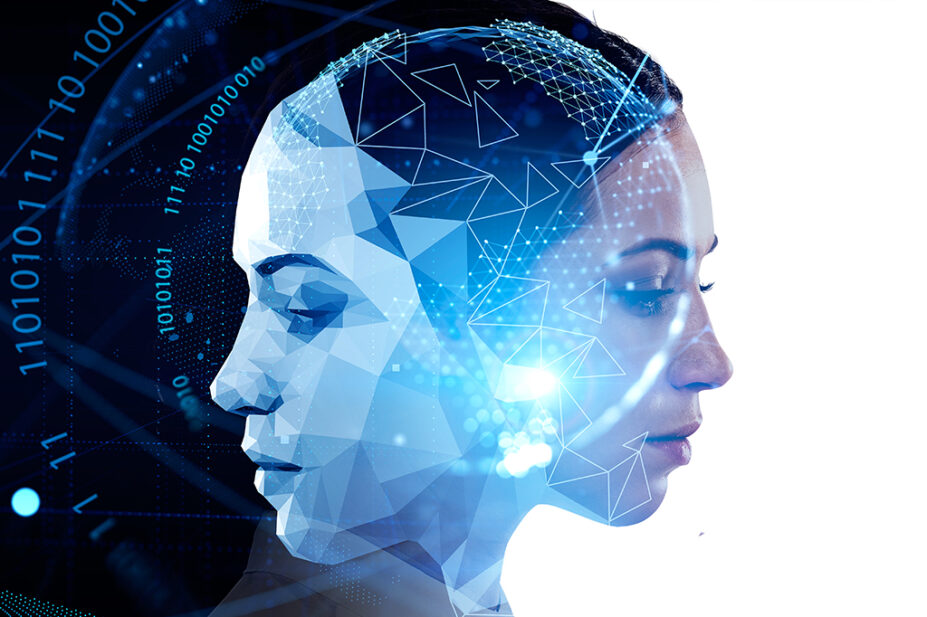
ismagilov
Imagine if next time you consult with a patient, you have an expert in the relevant field sat next to you, scouring the latest scientific literature, combing through millions of case studies in seconds, and applying this knowledge in an instant to help you provide the best possible care. Sounds too good to be true? It may not be as far-fetched as it seems, or as far away. But it won’t be a real-life expert, it will be your virtual artificial intelligence (AI) assistant — in the form of the next generation of clinical decision support tools.
Reliance on traditional guidelines or rule-based systems are a “big problem in clinical decision support”, explains Tim Rawson, National Institute for Health and Care Research Academic Clinical Fellow in Infectious Diseases, since patients are “a lot more complex than this”.
Rawson’s research team at Imperial College London is developing an artificial intelligence (AI) clinical decision support system (CDSS) called EPIC-IMPOC (Enhanced, personalised and integrated care for infection management at point of care), an infection management interface that supports clinical decision making, at the bedside, throughout patients’ treatment.
“EPIC-IMPOC gives the clinician targeted information on infection management,” says Richard Wilson, the team’s research pharmacist. It employs AI in the form of two algorithms: a machine learning algorithm to predict the likelihood of an infection and a ‘case-based reasoning’ algorithm that provides a recommendation of antimicrobial selection and dosage. “Case-based reasoning matches each patient’s biochemistry with a patient in the database, what they had been treated with, and whether that had been successful or not.”
This automated analysis comparing patient trajectories with thousands of past patient case data also supports treatment optimisation, such as intravenous to oral switching or treatment cessation. Avoiding issues of accurate recall and giving access to learnings equivalent to years of clinical experience, these systems could bring serious improvements to patient care.
There’s a big opportunity to provide these non-infection specialist doctors some sort of assistance in diagnosing infection
Richard Wilson, research pharmacist, Imperial College London
“The people who are actually treating the majority of patients with infection are going to be non-infection specialists,” adds Wilson. “There’s a big opportunity there to provide these non-infection specialist doctors some sort of assistance in diagnosing infection.”
Ahead of moving to clinical trials, the team is also building compliance with over-arching policies, such as antimicrobial stewardship targets or cost-saving initiatives, into the algorithms for an additional breadth of function[1].
Another system in development that also acknowledges the complexity of the modern patient is the IACT (International Anticholinergic Cognitive Burden Tool),3 a web-based CDSS that uses AI to score drugs for anticholinergic risk.
“My main pitch point is this is going to help people on polypharmacy,” says Chris Fox, professor of clinical psychiatry at the University of Exeter and lead of the IACT project. “People who have multiple conditions, those are the people at most risk of damage [from] medications.”
While there are several anticholinergic burden tools already in use, they are static datasets that have not been updated since they were developed over a decade ago. “In essence, the tools are not fit for purpose,” Fox claims.
IACT uses a ‘natural language processing’ machine learning technique, which is able to ’read’ drug databases for key words and phrases indicative of anti-muscarinic activity[2]. The tool combines this with a read of the drug’s chemical structure for anticholinergic potential to derive an overall risk. Early, unpublished tests show a 15% increased sensitivity in assessing anticholinergic risk compared with current anticholinergic CDSS, and an improved accuracy in predicting falls in patients with high anticholinergic loads.
They’re not meant to be taking over decision making for clinicians
Tim Rawson, National Institute for Health and Care Research Academic Clinical Fellow in Infectious Diseases
The tool is able to dynamically keep up to date with new literature and new drugs, but the team is not stopping there, looking to add more layers of wrap-around functionality.
“Pharmacists have asked us: can we get some safe dosing on here?” Fox adds. “Ultimately, yes. But, at the moment, it will [only] say, from the literature, 20mg of olanzapine is more anticholinergic than 5mg.”
“And there is an added option to bring in monitoring — a system that interrogates the patient, if they are on a drug, and says, ‘Mrs Smith, you’re on these drugs, have you had any of these symptoms?’ And that then feeds back and gives you an alert, which you can read and go, hang on a minute, well there’s some warning symptoms of anticholinergic.”
EPIC-IMPOC and IACT are typical of the next generation of CDSSs (see Table)[3–12].
Leveraging data
Increasingly, CDSSs are evolving away from ‘knowledge-based’ systems deriving rules from guidelines and expert knowledge. Instead, ‘non-knowledge-based’ CDSSs use large datasets of patient case data and analyse their treatment trajectories, applying advanced AI methods to create rules, or ‘algorithms’, to achieve optimal patient outcomes[13].
Several AI techniques can be applied, but popular methods involve machine learning — a sub-set of AI where the algorithm is ‘trained’ on datasets to optimise performance. The resulting fine-tuned algorithm holds the learnings of thousands or even hundreds of thousands of previous cases and can apply these insights at each decision point in a decision-making pathway.
The ability of AI to leverage data like this means AI CDSSs can take a broader view of treatment pathways than previous CDSSs, as well as the human clinician.
But the unanimous opinion is that AI CDSSs are still just tools (see Box).
Box: Five ways artificial intelligence might help pharmacists in future
- Independent prescribing support — with pharmacists set to be independent prescribers at the point of registration by 2026, artificial intelligence (AI) clinical decision support system (CDSS) can help newly qualified pharmacists build skills with confidence by providing prescribing recommendations or monitoring for medication errors;
- Everyone an expert — similarly, AI CDSS can also improve standards by supporting clinicians working outside their specialism, providing additional safety nets to prevent treatment errors;
- Empowered patients — AI CDSS can be embedded into wearable devices, closed-loop systems, or user-friendly apps, freeing up healthcare professionals’ time;
- More integrated care — AI CDSSs are typically linked to electronic health records, allowing for remote monitoring of medicines adherence or clinical alerts, supporting care in the community;
- Day-to-day drudgery — similarly to dispensing robotics, AI can also take on a pharmacy’s more mundane tasks; improving inventory management by predicting stock shortages, optimising staffing schedules, or automating deliveries, letters and emails.
Rawson is clear: “They’re not meant to be taking over decision making for clinicians.
“They are just meant to be providing better, new, real-time and accurate information from which the clinician can make that decision.”
Which is just as well, really, because — as with most AI applications — they lack street smarts.
Dirty data
One AI model attempting to predict pneumonia mortality risk was found to be assigning patients with asthma as low risk[14]. But this was because asthma patients were more likely to be admitted to the intensive care unit where they received more comprehensive treatment, which mitigated some of their risk, not because of any factor inherent to the biology of the condition.
And there are more insidious problems that AI, as any data-based tool, is prone to — biased or so-called ‘dirty’ data.
“If bias is ingrained in the dataset, and you train the algorithm on this dataset, the bias would be replicated, and [it] has the risk of being replicated at [a] large scale,” explains postdoctoral research associate Baptiste Vasey, whose research at the University of Oxford focuses on computer-aided decision support to improve the management of patients presenting with postoperative complications.
A serious example might be the false but prevalent assumption in Western healthcare that black patients feel less pain[15–17].
“If an algorithm learns the correlation between this demographic and the fact of receiving less treatment, due to pre-existing bias, then the next time the algorithm sees someone from this demographic at hospital,” Vasey warns, “it will prescribe less painkiller or a different type of treatment.”
For reasons such as this, all AI-CDSSs need scrutiny and testing before they are used on live patients.
You can’t really defend yourself by saying, well the algorithm told me to do that
Baptiste Vasey, postdoctoral research associate, University of Oxford
The development pipeline that aims to achieve this starts with in silico evaluation, where the initial training dataset is divided, so one part can be used to build and optimise the algorithm, while the other can test its accuracy. The threshold of accuracy is very high — typically held up against the accuracy of the expert in that field.
Then, the algorithm undergoes further rounds of validation and improvement with fresh data from a range of different settings deemed relevant to its intended use, before being tested against prospective clinical data for a preliminary read on its impact and safety. Finally, traditional randomised controlled trials are employed. However, although the algorithm’s impact on patient outcomes can be compared against a control arm, evaluation of its efficacy is unavoidably linked to successful clinician interaction with the system.
Known as ‘the human factor’, a new reporting framework DECIDE-AI (Developmental and Exploratory Clinical Investigations of Decision support systems driven by Artificial Intelligence) places emphasis on figuring these kinks out in early-stage clinical trials.
“Analyse how the system interacts with the workflow and the people,” Vasey, lead author of the DECIDE-AI proposal, emphasises[18]. “If you do that at the early stage, you have more chances to tweak your system, to update your system, than if you do it at a later stage.”
Akin to phase I and II drug trials, DECIDE-AI addresses safety and human factors — both practicalities, such as usability or integration into clinical workflow, and social elements, such as bias.
But the AI element of CDSSs introduces another problem — clinician trust in the system.
“You can’t really defend yourself by saying, well the algorithm told me to do that,” Vasey adds. “No, you’re the doctor, you take responsibility for the patient.”
One approach that could tackle trust, usability and bias together is ‘explainability’.
Blackbox AI
“We have two types of explanations: global and local,” Yuhan Du, a postdoctoral research fellow at University College Dublin, describes. “With global explanation, we can tell which factors are having effects on the outcomes, so we can tell if race has been a factor. With local, you can tell which factor is playing an effect [on the system’s recommendation] for each patient.” Both types explain how the AI algorithm works.
Some AI-CDSSs are straight-forward enough to understand and naturally more explainable. For instance, case-based reasoning algorithms, which match a presenting case to similar ones in its database as the basis of their recommendations, work strikingly similarly to how an expert clinician might consult the literature or wrack their brain for memories of past cases.
These are very complex algorithms and we do not know how they work
Yuhan Du, postdoctoral research fellow, University College Dublin
The real issue is ‘blackbox AI’.
“These are very complex algorithms,” Du explains, and we do not know how they work.
Mostly using machine learning techniques, blackbox AI is fed data and told ‘go’, given only the often high-level end ‘goal’ for direction, such as reduce hospital stay duration. While prioritising outputs this way capitalises on the strengths of AI in making connections humans cannot, it gives developers less control over how it reaches that ‘goal’, exposing it to bias, error and reduced user-trust as a result. Developers can try to reverse engineer its decision pathway, analysing how the patient datapoints intersect to generate a recommendation, but this is difficult given the amount of datapoints involved.
Even when understandable, how this is presented to the user can also impact clinician trust. This could be as simple as presenting a probability as 80% instead of 0.8 or experimenting with different visualisations of patient data — perhaps incorporating textual narrative to highlight contributing factors.
Explainability can take many forms, but working it out early in the pipeline can make larger trials, regulatory approval and implementation smoother to navigate.
These are perhaps the final hurdles for AI-CDSSs. Regulation remains an evolving field; the Medicines and Healthcare products Regulatory Agency (MHRA) generally considers AI-CDSSs as medical devices, which are registered but not directly approved by the MHRA in the UK[19–21]. And while there are some AI-CDSSs currently registered with the MHRA, these are mostly imaging diagnostics, which is an advanced area of AI healthcare.
Implementation is also more challenging in the UK.
Despite the UK having a head-start over most of the world in terms of widespread digitisation and electronic health record use, “access to the NHS is very difficult”, says Bernard Hernandez, research associate at the Bio-Inspired Technology Centre, Imperial College London, of his experiences attaining data for the EPIC-IMPOC system. And with each local health board or integrated care system following local policies, data and clinical workflows are not standardised across the UK, making commercialisation tricky. “They say the NHS is a very difficult market to penetrate.”
The government released its National AI strategy in 2021, laying out a ten-year vision to ‘AI-enable’ the economy and position the UK as a global leader in AI, charging the national Office for AI to deliver this mission[22]. Part of this is the NHS AI Lab, which is creating a national strategy for AI in health and social care, and working on developing and implementing AI healthcare solutions[23]. The government also launched an AI white paper in March 2023, which sets out a new approach to regulating AI in the UK, with the aim of driving responsible innovation while maintaining public trust[24].
The outlook for AI CDSSs is positive, hopeful — but cautious.
“In other areas — [the chatbot] ChatGPT or [board game playing] AlphaGo — there are applications of AI that have proven useful. But in medicine, the evidence of real impact is quite scarce at the moment,” Vasey warns. “We as a field would need to produce this evidence, and relatively soon, if we want to keep the interest of funders, of scientists and of the public.”
- 1EPIC-IMPOC. Imperial College London. https://www.imperial.ac.uk/bio-inspired-technology/research/infection-technology/epic-impoc/ (accessed 31 Mar 2023).
- 2Secchi A, Mamayusupova H, Sami S, et al. A novel Artificial Intelligence-based tool to assess anticholinergic burden: a survey. Age and Ageing. 2022;51. doi:10.1093/ageing/afac196
- 3Chiang S, Rao VR. Choosing the Best Antiseizure Medication—Can Artificial Intelligence Help? JAMA Neurol. 2022;79:970. doi:10.1001/jamaneurol.2022.2441
- 4Qassim S, Golden G, Slowey D, et al. A Mixed-Methods Feasibility Study of a Novel AI-Enabled, Web-Based, Clinical Decision Support System for the Treatment of Major Depression in Adults. 2022. doi:10.1101/2022.01.14.22269265
- 5Liu S, See KC, Ngiam KY, et al. Reinforcement Learning for Clinical Decision Support in Critical Care: Comprehensive Review. J Med Internet Res. 2020;22:e18477. doi:10.2196/18477
- 6The AI Clinician. Imperial College London. https://www.imperial.ac.uk/artificial-intelligence/research/healthcare/ai-clinician/ (accessed 31 Mar 2023).
- 7Komorowski M, Celi LA, Badawi O, et al. The Artificial Intelligence Clinician learns optimal treatment strategies for sepsis in intensive care. Nat Med. 2018;24:1716–20. doi:10.1038/s41591-018-0213-5
- 8Festor P, Jia Y, Gordon AC, et al. Assuring the safety of AI-based clinical decision support systems: a case study of the AI Clinician for sepsis treatment. BMJ Health Care Inform. 2022;29:e100549. doi:10.1136/bmjhci-2022-100549
- 9Corny J, Rajkumar A, Martin O, et al. A machine learning–based clinical decision support system to identify prescriptions with a high risk of medication error. Journal of the American Medical Informatics Association. 2020;27:1688–94. doi:10.1093/jamia/ocaa154
- 10Damiani G, Altamura G, Zedda M, et al. Potentiality of algorithms and artificial intelligence adoption to improve medication management in primary care: a systematic review. BMJ Open. 2023;13:e065301. doi:10.1136/bmjopen-2022-065301
- 11Syrowatka A, Song W, Amato MG, et al. Key use cases for artificial intelligence to reduce the frequency of adverse drug events: a scoping review. The Lancet Digital Health. 2022;4:e137–48. doi:10.1016/s2589-7500(21)00229-6
- 12Tan S-B, Kumar KS, Gan TRX, et al. CURATE.AI – AI-derived personalized tacrolimus dosing for pediatric liver transplant: A retrospective study. 2022. doi:10.1101/2022.11.24.22282708
- 13Sutton RT, Pincock D, Baumgart DC, et al. An overview of clinical decision support systems: benefits, risks, and strategies for success. npj Digit. Med. 2020;3. doi:10.1038/s41746-020-0221-y
- 14Du Y, Antoniadi AM, McNestry C, et al. The Role of XAI in Advice-Taking from a Clinical Decision Support System: A Comparative User Study of Feature Contribution-Based and Example-Based Explanations. Applied Sciences. 2022;12:10323. doi:10.3390/app122010323
- 15Hoffman KM, Trawalter S, Axt JR, et al. Racial bias in pain assessment and treatment recommendations, and false beliefs about biological differences between blacks and whites. Proc. Natl. Acad. Sci. U.S.A. 2016;113:4296–301. doi:10.1073/pnas.1516047113
- 16Iacobucci G. Most black people in UK face discrimination from healthcare staff, survey finds. BMJ. 2022;:o2337. doi:10.1136/bmj.o2337
- 17Morden NE, Chyn D, Wood A, et al. Racial Inequality in Prescription Opioid Receipt — Role of Individual Health Systems. N Engl J Med. 2021;385:342–51. doi:10.1056/nejmsa2034159
- 18Vasey B, Nagendran M, Campbell B, et al. Reporting guideline for the early-stage clinical evaluation of decision support systems driven by artificial intelligence: DECIDE-AI. Nat Med. 2022;28:924–33. doi:10.1038/s41591-022-01772-9
- 19MHRA. Software and AI as a Medical Device Change Programme – Roadmap. Medicines and Healthcare products Regulatory Agency. 2022.https://www.gov.uk/government/publications/software-and-ai-as-a-medical-device-change-programme/software-and-ai-as-a-medical-device-change-programme-roadmap (accessed 31 Mar 2023).
- 20MHRA. Good Machine Learning Practice for Medical Device Development: Guiding Principles. Medicines and Healthcare products Regulatory Agency. 2021.https://www.gov.uk/government/publications/good-machine-learning-practice-for-medical-device-development-guiding-principles/good-machine-learning-practice-for-medical-device-development-guiding-principles (accessed 31 Mar 2023).
- 21MHRA. Guidance: Medical device stand-alone software including apps (including IVDMDs). Medicines and Healthcare products Regulatory Agency. https://assets.publishing.service.gov.uk/government/uploads/system/uploads/attachment_data/file/1105233/Medical_device_stand-alone_software_including_apps.pdf (accessed 31 Mar 2023).
- 22Department for Business, Energy & Industrial Strategy, Department for Business, Energy & Industrial Strategy, Department for Science, Innovation & Technology, et al. National AI Strategy. Gov.uk. 2022.https://www.gov.uk/government/publications/national-ai-strategy (accessed 31 Mar 2023).
- 23The NHS AI Lab. NHS England. https://transform.england.nhs.uk/ai-lab/ (accessed 31 Mar 2023).
- 24A pro-innovation approach to AI regulation. Gov.uk. 2023.https://www.gov.uk/government/publications/ai-regulation-a-pro-innovation-approach/white-paper (accessed 3 Apr 2023).